

In most cases, image thresholding and data extraction is still laborious and requires manual input. Although image acquisition is relatively straightforward, image analysis is plagued by a number of bottlenecks. Image analysis pipelines for easy phenotyping have recently become more widely available such as those for measuring leaf area, leaf growth and root traits ( Bours et al., 2012 Easlon & Bloom, 2014 Seethepalli et al., 2021). Therefore, developing reliable and efficient methods for automated extraction of phenotype data from plant images is crucial. However, manual analysis of these image datasets can also be time-consuming, inconsistent, and requires expert observers.

Analysis of such image datasets could allow for a more detailed understanding of dynamic developmental changes and for phenotypic traits to be measured in a non-destructive manner in comparison to current commonly used manual phenotyping methods. The need to better characterize plant developmental growth stages and monitor traits that affect yield has led to an increased demand for and collection of high-throughput, high resolution plant image datasets ( Costa et al., 2019). By obtaining insights on how genetics and environmental pressures lead to different phenotypic response in plants, more suitable and sustainable crops can be selected for growth in specific environments, as by identifying the genetic basis of phenotypic variation a better understanding of the factors driving plant adaptation and stress tolerance could be achieved. For example, studies such as Genome-Wide Association Studies (GWAS) involve testing genetic variants across genotypes of a population to identify genotype-phenotype associations and provide essential information for plant breeding ( Brachi et al., 2011 Alseekh et al., 2021). With the recent rapid progression in functional genomics due to advances in high throughput sequencing, quantitative analyses of plant traits are of increasing relevance as it could allow for the links between genotype and phenotype to be explored in greater depth. Identifying connections between plant genotype and phenotype is essential to advance our understanding of underlying developmental mechanisms in plant biology. The study of plant traits, such as plant architecture, growth, development, physiological or biochemical profiles is known as plant phenotyping. There is also potential for the fine-tuned Stardist-3D method to be applied to imagery of seeds from other plant species, as well as imagery of similarly shaped plant structures such as beans or wheat grains, provided the structures targeted for detection and segmentation can be described as star-convex polygons. Additionally, the fine-tuned StarDist-3D provides an efficient way to create a dataset of segmented images of individual seeds that could be used to further explore the factors affecting seed development, abortion and maturation synchrony within the pod. Results: With a relatively minimal training effort, this fine-tuned StarDist-3D model accurately detected (Validation F1-score = 96.3%,Testing F1-score = 99.3%) and predicted the shape (mean matched score = 90%) of seeds.ĭiscussion: This method then allowed rapid extraction of data on the number, size, shape, seed spacing and seed location in specific valves that can be integrated into models of plant development or crop yield. Methods: In this paper, a current gold standard computed vision method for detecting and segmenting objects in three-dimensional imagery (StartDist-3D) is applied to X-ray micro-computed tomography scans of oilseed rape ( Brassica napus) mature pods. Therefore, the development of efficient and reliable machine learning methods for extracting phenotype data from plant imagery is crucial. However, manual data extraction from such datasets are known to be time-consuming and resource intensive. Introduction: Plant image datasets have the potential to greatly improve our understanding of the phenotypic response of plants to environmental and genetic factors. 3Department of Chemical Engineering and Biotechnology, School of Technology, University of Cambridge, Cambridge, United Kingdom.2Department of Plant Sciences for the Bioeconomy, Rothamsted Research, Harpenden, United Kingdom.
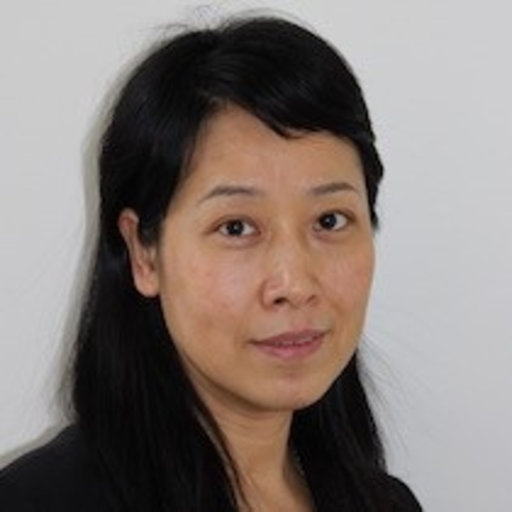
1Environment and Sustainability Theme, AI for Science and Government Programme, The Alan Turing Institute, London, United Kingdom.Evangeline Corcoran 1* Laura Siles 2 Smita Kurup 2 Sebastian Ahnert 1,3
